Quantifying Cognitive Process
Science and Peer Reviewed Journal Publications
Literature Review through 2024
High Accuracy in All Stages of Memory Impairment.
For over 20 years of testing and development, the MCI Screen has high overall accuracy, sensitivity and specificity in distinguishing normal aging from all stages of memory impairment from Normal to MCI to Dementia.
Source: Proceedings of National Academy of Science. 2005; 102(13):4919-24.
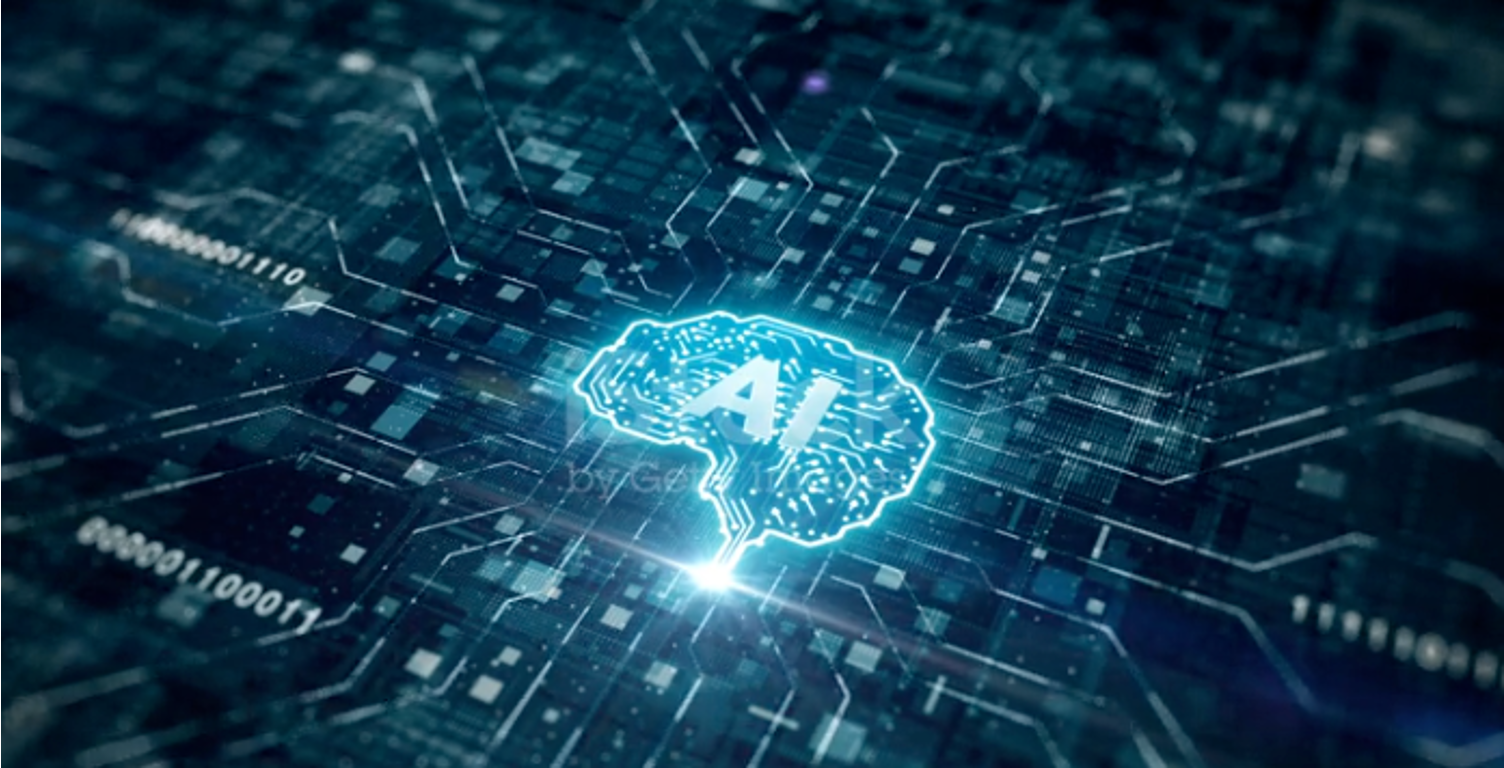
MCI Screen: An AI solution predicting the onset of Alzheimer's Symptoms
MCI Screen detects cognitive changes that precede perceptible cognitive decline in people that may with Alzheimer's Disease.
Our Mission: Quantify Cognitive Processes Through Advanced Research
High Accuracy in All Stages of Memory Impairment.
For over 20 years of testing and development, the MCI Screen has high overall accuracy, sensitivity and specificity in distinguishing normal aging from all stages of memory impairment from Normal to MCI to Dementia.
MCI Screen predicts probability of impairment vs. normal, accuracy of 97% (C.I. 97–98%), sensitivity of 94% (C.I. 93–95%), and specificity of 89%.
Methods to improve the detection of mild cognitive impairment.
Shankle WR, Romney AK, Hara J, Fortier D, Dick MB, Chen JM, Chan T, Sun X. Proceedings of the National Academy of Sciences. 2005 Mar 29;102(13):4919-24.
Early Detection of AD with MCI Screen vs MMSE vs Clock Drawing. Overall accuracy = {96%, 66%, 54%. The MMSE and CDT were not valid for early detection, while MCI Screen had high validity and accuracy in the primary care cohort.
Detecting cognitive impairment in primary care: performance assessment of three screening instruments.
Trenkle DL, Shankle WR, Azen SP. Journal of Alzheimer’s Disease. 2007 May 29;11(3):323-35.
MCI Screen: More Accurate than Commonly Used Assessments
The MCI Screen has high accuracy compared to commonly used assessments in physicians’ offices. In fact, no other instrument in the published literature can reliably distinguish MCI from Normal, whereas the MCI Screen does so with 97.3% accuracy.
Precise evaluation of cognitive function can facilitate deep insight into overall brain health, which is affected by a variety of factors including normal aging, neurodegenerative disorders and their severity, pharmaco and non-pharmacotherapies, and many others.
As a framework for evaluating the directly
observable aspects of cognition, the Diagnostic and Statistical Manual of Mental Disorders (DSM–5) specifies 6 key cognitive domains (Figure 1),1 each consisting of several subdomains.
However, much deeper insight can be gained by characterizing underlying processes of encoding and retrieval that cannot be directly observed. Embic’s quantified cognitive processes (qCPs) represent such underlying
factors and enable more precise evaluation of cognitive health.
DEVELOPMENT AND VALIDATION OF QUANTIFIED COGNITIVE PROCESSES (qCP)
Generating Comparable qCPs Across Different WLM Tests
To evaluate the generalizability of qCPs across different WML tests, values were generated using item
response data from 2,456 subjects who were assessed with three different WLM tests (i.e., ADAS-Cog,
MCI Screen, or AVLT) and had diagnoses of cognitively normal, amnestic MCI, or AD dementia.
The changes in qCPs from normal to AD dementia were consistent across the three different WLM tests
and data sources (Figure 3). The results demonstrate that these qCPs are robust and generalizable,
regardless of the underlying test protocol, and allow comparison of various studies conducted using
different WLM tests.
Detecting Cognitive Changes that Precede Perceptible Cognitive Decline in Subjects with AD
To evaluate the ability of qCPs to predict impending cognitive decline due to AD, values were
generated from baseline assessments of 640 subjects who were assessed longitudinally with the AVLT
WLM test in the Mayo Clinic Aging Registry.
While all subjects were considered, according to best practice industry standards, to have normal cognitive function at baseline, the qCPs clearly distinguished the group who would maintain normal cognition (stable) from the group that would progress to aMCI or AD dementia (progressor) within three years. This was further validated with baseline assessments of 503 subjects who were assessed with the ADAS-Cog WLM test in the
Alzheimer’s Disease Neuroimaging Initiative (ADNI).*
The results showed, despite both stable and progressor groups being classified as cognitively healthy at baseline ADNI measurement, progressors already had measurable deficits in unobservable processes of encoding and retrieval (Figure 4).These studies clearly demonstrate the ability of qCPs to pragmatically identify very early cognitive changes in subjects who are in the pre-symptomatic stages of AD. Furthermore, Embic’s qCPs could
potentially serve as important outcome measures in trials for therapies that effect cognition.
* This study was supported by NIH SBIR grant#: 1R44AG065126.
Predicting clinical decline over 36 months with a memory-based digital biomarker
Davide Bruno, Ainara Jauregi Zinkunegi, Jason R Bock
First published: 25 December 2023
Background
Word-list recall tests are routinely employed in clinical practice for dementia screening. Process scoring and latent modeling of these tests, where underlying neurocognitive mechanisms are exploited, have shown potential to enhance test accuracy without requiring test redesign. We examined Embic’s digital biomarker M, a measure of recall derived from multinomial processing trees and hierarchical Bayesian computational methods, as a predictor of conversion to mild cognitive impairment (MCI) and Alzheimer’s disease (AD), and of increase in Clinical Dementia Rating (CDR) score.
Methods
Secondary data analyses were carried out from ADNI data. M was computed from Rey’s AVLT data, and compared to standard AVLT clinical metrics (total and delayed recall). We conducted logistic regression analyses with diagnosis at 36 months (normal vs. MCI + AD) as outcome; all 169 participants were classified as normal at baseline, and 13 of these progressed to a clinical diagnosis (see Table 1). We controlled for baseline age, gender and years of education; and ran separate analyses with other metrics to avoid multicollinearity. We then repeated the same analytical framework with CDR scores (stable at 0 vs. increased to 0.5 or 1) at 36 months as outcome: stable participants were 141, and 24 increased (see Table 2). Covariates and predictors were the same as in the previous analysis.
Results
M predicted conversion to MCI or AD after three years (FDR-corrected p value = 0.048), while neither AVLT total nor delayed recall did. Performance metrics showed that M generated 92% accuracy, or probability of yielding a correct classification, and 100% specificity, or no false positives (Figure 1). Analogously, M, but not standard AVLT metrics, also predicted a CDR increase after three years (FDR-corrected p value = 0.003), with high accuracy (84%), specificity (96%), and precision (80%), or positive predictive value (Figure 2). Sensitivity analyses with ordinal regression broadly confirmed these findings.
Conclusions
These results indicate Embic’s digital biomarker M outperforms some of AVLT’s traditional metrics in identifying individuals who will progress to MCI and AD after three years, from a healthy baseline.
Speak with your Account Representative to set up your account.
Learn how many underlying medical conditions can cause mild cognitive impairment, and many are treatable.
Learn how to work with your physician can determine the underlying cause and provide a treatment plan.
Learn how to detect dementia early, and understand why is very important because in many cases, it can be diagnosed and treated by a physician to stop or delay the decline in mental function for years.
References: Development of and Validation of the Embic Quantified Cognitive Processes
- 1. Sachdev PS, Blacker D, Blazer DG, Ganguli M, Jeste DV, Paulsen JS, Petersen RC. Classifying neurocognitive disorders: the DSM-5 approach. Nat Rev Neurol. 2014 Nov;10(11):634-42. doi: 10.1038/nrneurol.2014.181. Epub 2014 Sep 30. PMID: 25266297.
- 2. Crane PK, Carle A, Gibbons LE, Insel P, Mackin RS, Gross A, et al. Alzheimer’s Disease Neuroimaging Initiative. Development and assessment of a composite score for memory in the Alzheimer's Disease Neuroimaging Initiative (ADNI). Brain Imaging Behav. 2012;6(4):502-16. doi: 10.1007/s11682-012-9186-z. PMID: 22782295; PMCID: PMC3806057.
- 3. Donohue MC, Sperling RA, Salmon DP, Rentz DM, Raman R, Thomas RG, Weiner M, Aisen PS; Australian Imaging, Biomarkers, and Lifestyle Flagship Study of Ageing; Alzheimer’s Disease Neuroimaging Initiative; Alzheimer’s Disease Cooperative Study. The preclinical Alzheimer Page 7 of 7 cognitive composite: measuring amyloid-related decline. JAMA Neurol. 2014 Aug;71(8):961-70. doi: 10.1001/jamaneurol.2014.803. PMID: 24886908; PMCID: PMC4439182.
- 4. Wang J, Logovinsky V, Hendrix SB, Stanworth SH, Perdomo C, Xu L, Dhadda S, Do I, Rabe M, Luthman J, Cummings J, Satlin A. ADCOMS: a composite clinical outcome for prodromal Alzheimer's disease trials. Journal of neurology, neurosurgery, and psychiatry 2016;87(9):993–999. https://doi.org/10.1136/jnnp-2015-312383.
- 5. Buckley RF, Sparks KP, Papp KV, Dekhtyar M, Martin C, Burnham S, Sperling RA, Rentz DM. Computerized Cognitive Testing for Use in Clinical Trials: A Comparison of the NIH Toolbox and Cogstate C3 Batteries. J Prev Alzheimers Dis. 2017;4(1):3-11. doi: 10.14283/jpad.2017.1. PMID: 29188853; PMCID: PMC5726304.
- 6. Papp KV, Rentz DM, Maruff P, Sun CK, Raman R, Donohue MC, Schembri A, Stark C, Yassa MA, Wessels AM, Yaari R, Holdridge KC, Aisen PS, Sperling RA. The Computerized Cognitive Composite (C3) in an Alzheimer's Disease Secondary Prevention Trial. J Prev Alzheimers Dis. 2021;8(1):59-67. doi: 10.14283/jpad.2020.38. PMID: 33336226; PMCID: PMC7755110.
- 7. Lee MD, Bock JR, Cushman I, Shankle WR. An application of multinomial processing tree models and Bayesian methods to understanding memory impairment. Journal of Mathematical Psychology. 2020; 95, Article 102328. https://doi.org/10.1016/j.jmp.2020.102328 8. Shankle WR, Hara J, Bock JR, Fortier D, Mangrola T, Lee MD, Alexander GE, Batchelder WH, Petersen RC, Kremers W. Using Graphical Hierarchical Bayesian Cognitive Process Models Applied to Common Memory Tests to Predict AD Pathology within Normal Subjects. CTAD 2018. Poster Presentation. Barcelona, November 2018.
- 9. Shankle WR, Bock JR, Hara J, Mangrola T, Fortier D, Lee MD, Kremers W, Petersen RC. Measuring Latent Cognitive Processes to Detect the Earliest Changes in Alzheimer’s Disease. AAIC 2018. Poster Presentation. Chicago, July 2018.
- 10. Bock JR, Hara J, Fortier D, Lee MD, Petersen RC, Shankle WR. Application of Digital Cognitive Biomarkers for Alzheimer's Disease: Identifying Cognitive Process Changes and Impending Cognitive Decline. J Prev Alzheimers Dis. 2021;8(2):123-126. doi: 10.14283/jpad.2020.63. PMID: 33569557.
- 11. Bock JR, Lee MD, Hara J, Fortier D, Mangrola T, Shankle WR. Preliminary Study: Hierarchical Bayesian Cognitive Processing Model Classification of CSF and PET Amyloid Positivity and Negativity from Cognitive Assessment Data. Poster Presentation. Alzheimer’s Association’s International Conference, Los Angeles, July 2019.
References: Memory Screen Subjective Assessment
- Early identification of Alzheimer's disease and related dementias. Agency for Health Care Policy and Research. Clin Pract Guidel Quick Ref Guide. 1996;19:1-28.
- Shankle, W.R., Datta, P., and Pazzani, M. Machine Learning Methods and Dementia Diagnosis. Alzheimer's Research, 2:95-99 (1996).
- Shankle, W.R., Mani, S., Pazzani, M. J. and Smyth, P. (1997). Use of a Computerized Subject Record Database of Normal Aging and Very Mildly Demented Patients to Compare Classification Accuracies Obtained with Machine Learning Methods and Logistic Regression. Computing Science and Statistics, 29: 201-209.
- W.R. Shankle, Subramani Mani, Michael J. Pazzani, and Padhraic Smyth. "Dementia Screening with Machine Learning methods." In Intelligent Data Analysis in Medicine and Pharmacology, Eds. Elpida Keravnou, Nada Lavrac and Blaz Zupan, Kluwer Academic Publishers. (1997)
- DesRosiers G, Hodges JR, Berrios G. The neuropsychological differentiation of patients with very mild Alzheimer's disease and/or major depression. J Am Geriatr Soc. 1995;43:1256-63.
- Chapman LL, White DA, Storandt M. Prose recall in dementia. A comparison of delay intervals. Arch Neurol. 1997;54:1501-4.
- Larumbe R. Detection of early cases of Alzheimer's disease.Application of the CERAD neuropsychological test battery. Rev Med Univ Navarra. 1997;41:6-11.
- Schmand B, Walstra G, Lindeboom J, Teunisse S, Jonker C. Early detection of Alzheimer's disease using the Cambridge Cognitive Examination. Psychol Med. 2000;30:619-27.
- Bayley PJ, Salmon DP, Bondi MW, Bui BK, Olichney J, Delis DC, Thomas RG, Thal LJ. Comparison of the serial position effect in very mild Alzheimer's disease, mild Alzheimer's disease, and amnesia associated with electroconvulsive therapy. J Int Neuropsychol Soc 2000;6:290-8.
- Wang PN, Wang SJ, Fuh JL, Teng EL, Liu CY, Lin CH, Shyu HY, Lu SR, Chen CC, Liu HC. Subjective memory complaint in relation to cognitive performance and depression: a longitudinal study of a rural Chinese population. J Am Geriatr Soc 2000;48:295-9.
- McCleary, R., Mulnard, R.A., and Shankle, W.R. Reproductive Health Risks in Dementia: Evidence From the 1986 National Mortality Followback Survey. Alzheimer's Research (1996).
- Yaffe et al., Arch Neurol., 2002;59:378-384.
- Morris MC, Evans DA, Bienias JL, Tangney CC, Wilson RS. Vitamin E and cognitive decline in older persons. Arch Neurol. 2002;59:1125-32.
- Zandi PP, Anthony JC, Khachaturian AS, Stone SV, Gustafson D, Tschanz JT, Norton MC, Welsh-Bohmer KA, Breitner JC; Cache County Study Group. Reduced risk of Alzheimer disease in users of antioxidant vitamin supplements: the Cache County Study. Arch Neurol. 2004;61(1):82-8.
- Engelhart MJ, Geerlings MI, Ruitenberg A, van Swieten JC, Hofman A, Witteman JC, Breteler MM. Dietary intake of antioxidants and risk of Alzheimer disease. JAMA. 2002;287:3261-3.
- Breitner JC, Gau BA, Welsh KA, et al. Inverse association of anti-inflammatory treatments and Alzheimer's disease: initial results of a co-twin control study. Neurology. 1994:44:227-232.
- Andersen K, Launer LJ, Ott A, Hoes AW, Breteler MMB, Hofman A. do nonsteroidal anti-inflammatory drugs decrease the risk of Alzheimer's disease? Neurology. 1995;45:1441-1445.
- Stewart WF, Kawas C, Corrada M, Metter EJ. Risk of Alzheimer's disease and duration of NSAID use . Neurology. 1997;48:626-632.
- McGeer PL, McGeer E, rogers J, Sibley J. Anti-inflammatory drugs and Alzheimer's disease. Lancet. 1990;335:1037.
- Broe GA, Grayson DA, Creasey HM, Waite LM, Casey BJ, Bennett HP, Brooks WS, Halliday GM. Anti-inflammatory drugs protect against Alzheimer disease at low doses.
- Rozzini R, Ferrucci L, Losonczy K et al. Protective effect of chronic NSAID use on cognitive decline in older persons. J Am Geriatr Soc. 1996;44:1025-1029.
- Fassbender K, Simons M, Bergmann C, Stroick M, Lutjohann D, Keller P, Runz H, Kuhl S, Bertsch T, von Bergmann K, Hennerici M, Beyreuther K, Hartmann T. Simvastatin strongly reduces levels of Alzheimer's disease beta -amyloid peptides Abeta 42 and Abeta 40 in vitro and in vivo. 10: Proc Natl Acad Sci U S A 2001 May 8;98(10):5856-61.
- Rockwood et al., Arch Neurol., 2002. 59:223-7.
- Buxbaum JD, Cullen EI, Friedhoff LT. Pharmacological concentrations of the HMG-CoA reductase inhibitor lovastatin decrease the formation of the Alzheimer beta-amyloid peptide in vitro and in patients Front Biosci 2002;7:a50-9.
- Shankle WR, Hara J, Chan T, Chen JM, Germain R, Ortiz M. Computer-Assisted CERAD Battery For Early Stage Detection of Dementia. Conference Proceeding: 2003 APA Annual Meeting, San Francisco, CA, May 17-22, 2003.